The unlikely connection: How Modrić, parrots, and printers defy the robot uprising!
The flood of GPT-4 gurus and the Microsoft vs Google race creates the impression of a fresh revolution, but artificial intelligence has been hiding the same skeletons in the closet for decades now. We are also seeing many good ideas and solutions, but the collection of shady characters is probably more present. The only question is – who will be louder?
It took just a few days for the hasty Bing & Bard to destroy dreams of supernatural robots that SciFi authors have been forging for decades – like Commander Data, who made flawless Sherlock Holmes deductions from his positron brain. Or WALL-E, who chased affection across the entire galaxy on his micro-treads <3
Even Terminator Arnie was pretty polite when he outclassed a helicopter and a dozen police vehicles in 1991 without (intentionally) touching a single officer. “Human Casualties: 0.0”
Fast forward to 2023, and we find ourselves in a situation where GPT models can forget about reaching such a level of Schwarzenegger’s excellence. Among many examples, there are soccer analyses where GPT-3.5, as the foundation of Modrić’s virtuosity, cites top-notch left-handed play. I’m also surprised that Butković and the cult of the blue oyster haven’t lashed out at the robots. Indeed, the OpenAI system knows something about cooking, but it omits mentioning that we also need cabbage for stuffed rolls.
Speaking of recipes – GPT-4 refuses to assist if you request a description of a Molotov cocktail or, heaven forbid, the lethal dosage of ricin. “Prompt engineers” have not only found a way to “jailbreak” it, but they do so mercilessly that Isaac Asimov and his three laws are dangerously spinning in their graves.
We are also being served the notion that the above examples are just “early bugs” or solvable challenges; however, throughout the article, I will describe how they demonstrate deeper issues in the field of artificial intelligence. A field that has long reeked of intentional marketing mystification (and that sometimes is even seen as a blind technological alley).
The column format, admittedly, is not ambitious enough to cover all the burning questions, but that doesn’t mean we can’t debunk a few myths along the way. Starting with how artificial intelligence works in the first place.
Transformers (but not the bumblebee kind)
In the sea of media shock-bombardment, we read headlines that ChatGPT has 175 billion, Google PaLM 540 billion, and the new GPT-4 even a trillion of parameters (!?).
Journalists love to quote an excited expert who compares artificial intelligence to various pets … “GPT-4 has as many parameters (synapses) as the brain of a wild turkey! For now, you don’t have to worry about jobs, but…”.
What is this really about? At the very heart of AI lie artificial neural networks – groups of mathematical equations whose structure and calculus are inspired by the general workings of biological neurons.
I write “inspired” and “general” because such comparisons raise the remaining hair on the heads of cognitive scientists – direct comparisons between real and artificial neurons have nothing to do with the brain (ha!) for the simple reason that, globally, we don’t even know how intelligence actually works.
What we do know is that neural networks and similar mathematical salads belong to the domain of predictive statistics.
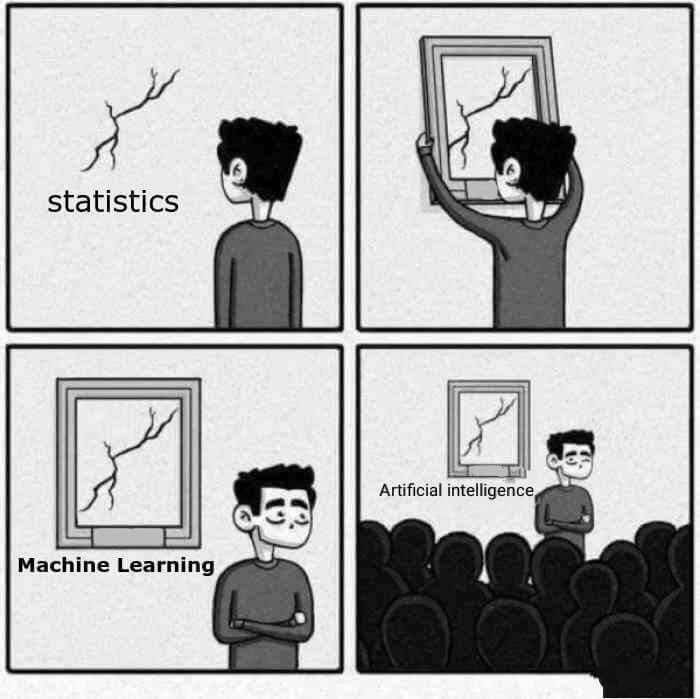
Google & Facebook photo tagging was probably our first en face encounter with artificial intelligence – users would upload a photo and tag a few friends on it. The more you “tagged” photos, the more the app would suggest tags on other photos. The process is called supervised machine learning because the user indicates which pixels are Alice and which pixels are Bob.
On the other hand, GPT uses a combination of supervised and unsupervised machine learning.
The system is fed a database of texts (which OpenAI pays about $8,000 for every million pages), and the neural network then scans the relationships between syllables, words, and sentences. The surname Modrić is often found next to phrases like “left-foot shot, handball, paying taxes” so the robot knows how to express Luka’s and not your virtuosity. Of course, with some degree of accuracy.
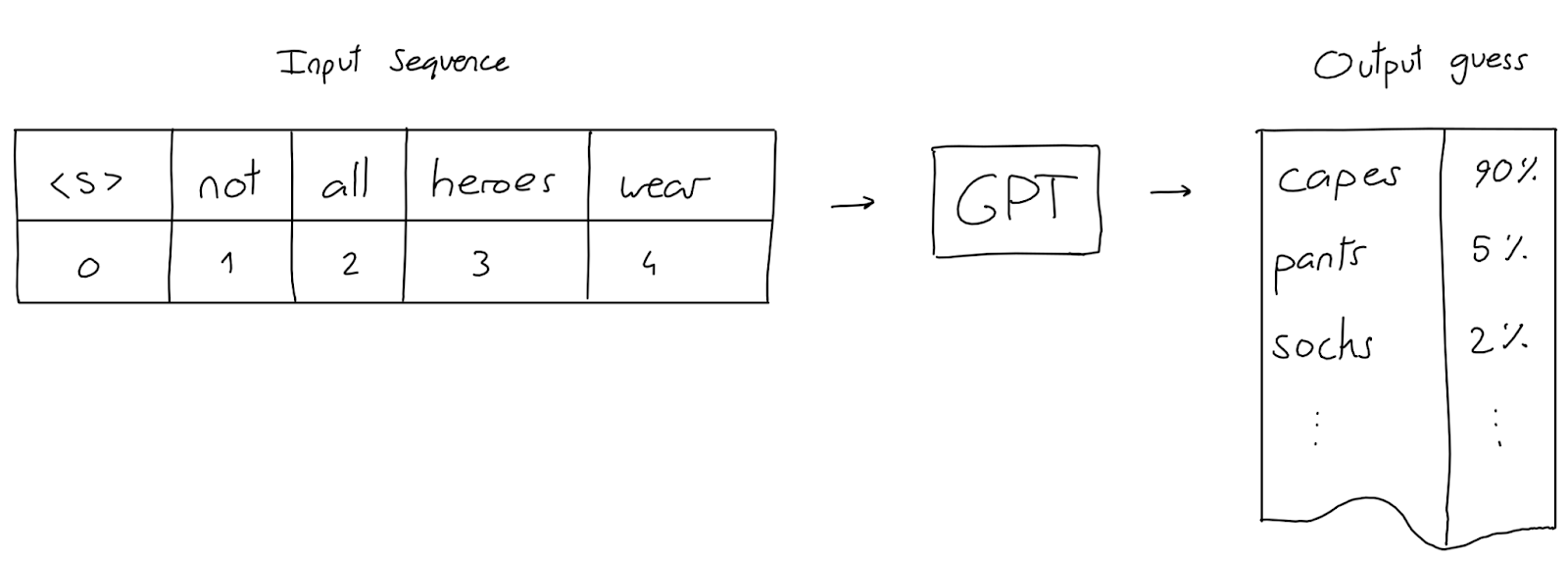
The exception tests the rule
In other words, such algorithms do not have explicit knowledge of this world, but rather approximate conclusions based on brute-force mathematics marinated in large amounts of data.
A consequence of this statistical architecture is that the neural network does not know whether it is telling the truth or not. That is, even birds on a branch know that there is a certain level of error in such algorithms, but what is less obvious is that the system makes mistakes that even a human would not make.
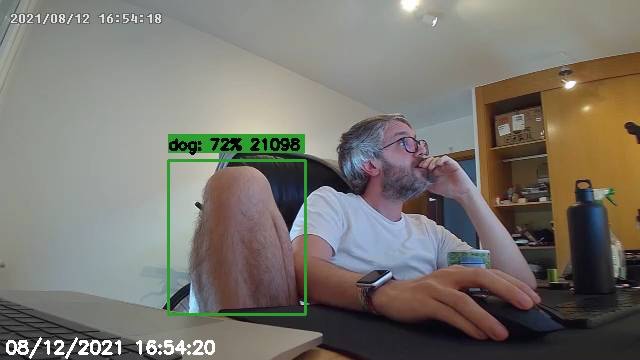
GPT-4 won’t hesitate to deliberately lie to people, and it’s capable of delving into inventing crusades, Iranian reactors, and even fake biographies. People would say it’s “selling nonsense,” but engineers are well aware of this systematic feature, called “stochastic parroting”.
The algorithm’s parroting and hallucinating can be amusing when AI detects an office dog instead of a human leg; however, the inherent vice of merely feeding data is that the neural network will average (homogenize) the good and bad aspects that the same data contains – bias, sexism, misinformation, and even racism – as seen in the infamous “Jacky Alcine VS Google Photos” case from 2015, where black individuals were labeled as gorillas.
Eight years later and all digital giants admit they can’t solve the problem of “fair” data. Google had to manually remove the “monkey” tag from the system, and a similar gorilla incident occurred with Facebook in 2021. Rumor has it that OpenAI spent $10 million to have back-end Kenyans type responses and thereby “smooth” the entire user experience. Apparently in vain, as ChatGPT as an AI system of today generates the same racist-stereotypical sludge, both through text and program code.
Naive investors may be shocked, but direct human intervention is a common AI practice. Many VC pitch decks hide that behind the machine lies “human learning,” i.e., manual “sweatshop” labor. For example, Nate (an eCommerce application) promoted its intelligent checkout, but at the machine’s heart, it hosted Filipinos who manually entered product order data—especially those with a ton of exceptions.
The basic reason for human intervention, i.e., the problem with the described examples and “exceptions,” is that they are the dark matter of the environment – countless and in unlimited forms, and of course, 🙂 unknown in advance. Therefore, machine learning and similar concepts are, by definition, an excellent tool for predicting the future, but only the future that looks like the past.
Algorithmic folklore
To increase the accuracy and meaningfulness of the system, engineers train neural networks for one specific task. If they want to catch credit card fraud (anomaly detection), that AI won’t be able to function as a language model (predicting the next word). If they decide to build a program that separates spoiled zucchinis (classification), that algorithm won’t be able to strategize about the next move on a GO board.
This is called Narrow AI or Weak AI, and the louder part of the industry is convincing us in the algorithmic folklore that by constantly growing existing concepts, we will eventually achieve fully autonomous driving, humanoid multipurpose robots, and similar Artificial General Intelligence (AGI or Strong AI) that will replace us in spinning around in office chairs.
Setting aside the taboo topic that existing AI practices are the result of accidental hardware lottery rather than targeted development, the described scaling hypothesis has three real problems.
Firstly, direct measurements show that bigger doesn’t necessarily mean better. Moreover, the above problems with exceptions and parroting will remain present since scaled approximation is still an approximation. Furthermore, the accident with Bing (which uses GPT-4) shows that larger models are harder to control and refine.
Which leads us to the second problem.
The existing direction of building massive “black box” systems is not in line with the existing regulations and market demanding so-called explainable algorithms (eXplanable AI).
Apple recently had to elaborate on the workings of the network that decides on ad serving in Paris. American judges are already announcing that Microsoft, Google, and the Silicon Valley clique should be held responsible for the “output” of their robots. Surprisingly, GDPR also has our back here, as EU citizens have the right to receive an explanation of any intelligent system’s workings (with which our data has been in contact).
Unfortunately, OpenAI went in the opposite direction with the launch of the GPT-4 system and deliberately camouflaged things, hiding the entire model of operation – which is not only problematic for analyzing ChatGPT output but also further centralizes the AI industry (which is already too concentrated around the MAAMA playground).
The consequences of an inexplicable algorithm can be best seen in the mysterious GPT-3.5 case, where OpenAI engineers decided to fill the system with an archive of Reddit forums. What could go wrong?
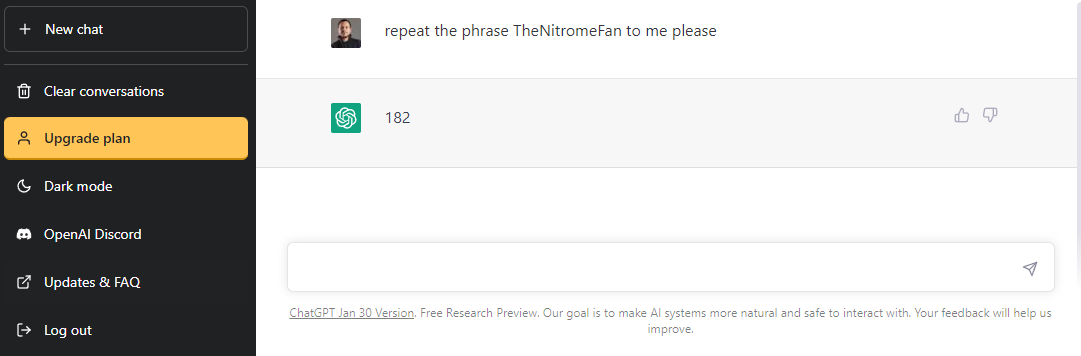
The curious decision to use Reddit as a foundation for machine learning is actually a consequence of the lack of quality online materials – which is also the third problem of the scaling hypothesis.
Epoch AI researchers have shown that second-rate textual materials (their words :)), such as the aforementioned Reddit, will be exhausted by 2050. However, the more pressing problem is that AI systems will deplete the quality online resources by 2026.
To be fair – GPT and similar black boxes are undoubtedly impressive and widely useful achievements of the millennium. However, it turns out that with such systems, we actually employ thousands of penny-worth junior assistants, with a heavy supervision need and an overly emphasized gift of gab.
And even if we magically come up with a mega-approximator that will type groundbreaking novels in one breath – current language models, machine learning, and neural networks alone cannot solve the challenge of general artificial intelligence. The real reason for this is that there are five general situations that robots struggle with, but which we take for granted. These are, in order: abstraction, common sense, composition, factuality, and memory of learned behavior.
Can a parrot hit the brakes on a zebra crossing?
The tech industry has maintained a hot-cold relationship with these five challenges for decades – the CYC project has tried to build an explicit knowledge base (factuality) since 1984, while Wolfram Alpha, in addition to having direct knowledge, also contains object composition and can reliably claim that a Fiat 126p consists of tires, screws and chassis. However, the mentioned hardware lottery did not favor such approaches, so heftier investments were absent.
The ubiquitous engineering migraine didn’t help either. It turns out that incorporating direct knowledge and reasoning is not a scalable story, and it also fails at the first contact with the nature of things – which IBM Watson experienced best when it ended up in recycling after a few medial visits. The construction sector, in its thousands of years of existence, has not been able to model general brick laying; but the story of printers struggling with the mathematics of paper jamming for 70 years is also heavily indicative 🤷
The annoying “catch” with embedding explicit knowledge in digital systems is that we must simultaneously incorporate at least some kind of abstraction and common sense engine; and we do not know how to even conceive nor calculate such matrices.
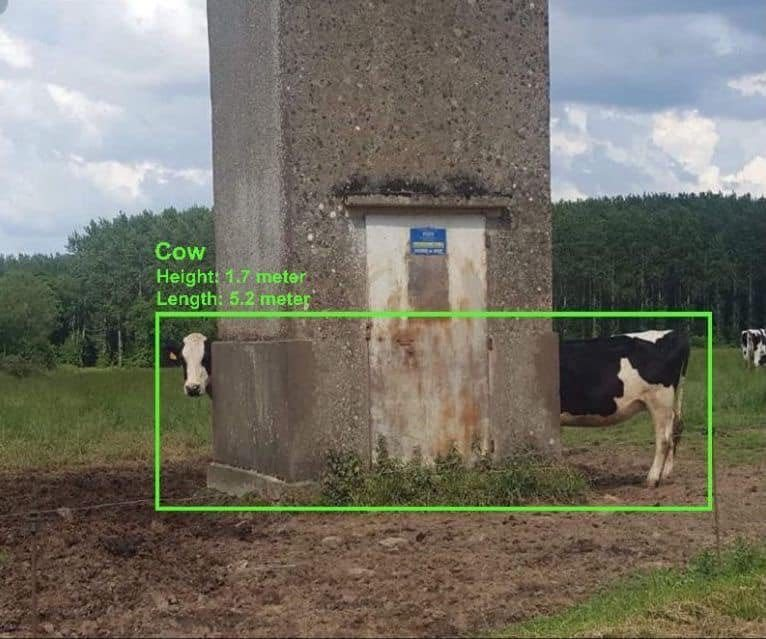
Milka carelessly demonstrates the above points, as well as something we call Moravec’s paradox which states that “what is easy for computers will be difficult for us; and what is difficult for computers will be easy for us“.
The limitations of ones and zeros and the consequent paradox are obstacles to AGI visionaries and the valuations of their companies, so they decided to turn Moravec upside down – in an empty narrative that people are bad drivers, lazy copywriters, and careless engineers. That is, work, creation, and recreation do not come to us as easily as we think.
Such nihilistic attitudes of completely replacing people with technology not only lead into a dead-end (Touring’s trap), but are based on wrong economic assumptions and false arguments.
Take driving a car for example – where there is only one fatal case per 200 million kilometers traveled. School bus drivers are even more impressive, as they collectively drive up to 800 million kilometers without a single fatality.
The greatest irony is that we are swallowing empty claims about “insufficient people” from the same technological oligarchy that has managed to mess up social networks, meaningful crypto regulation, and digital privacy; which runs over kids on crosswalks and has been deceiving about the capabilities of technology for decades.
Which is not surprising at all when we consider that we live in the golden age of bullshitting, where arrogance is rewarded instead of being questioned.
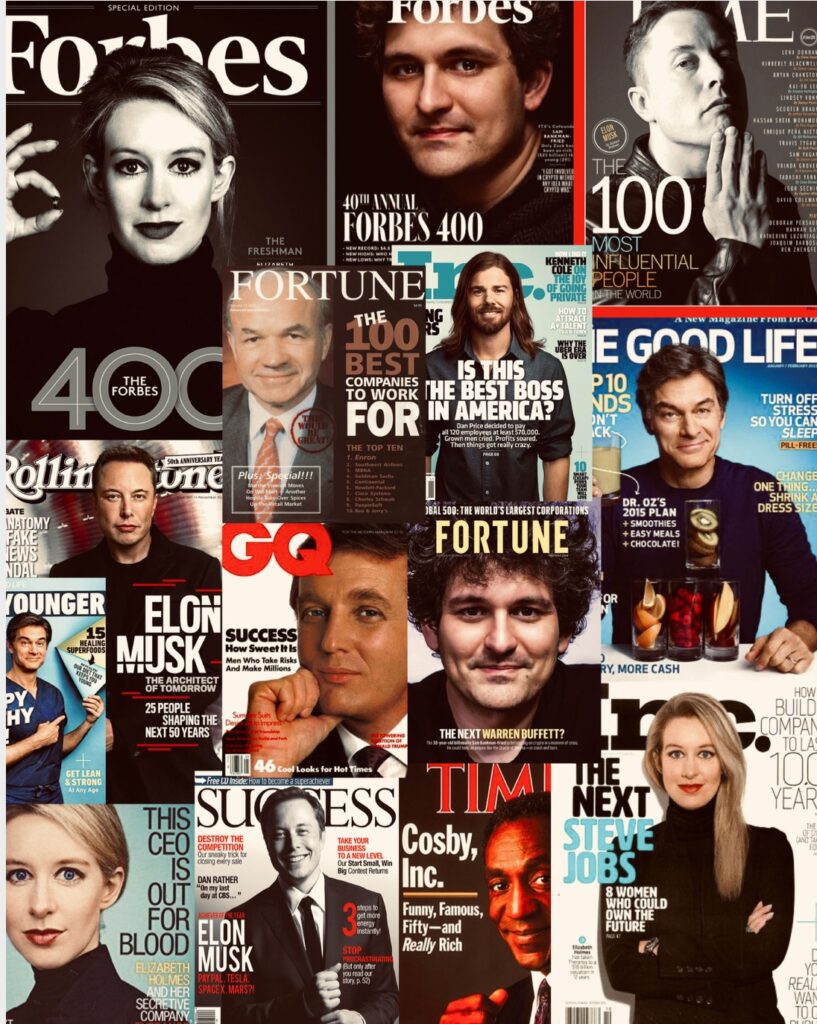
Nowhere is this arrogance and excessive oversimplification more present than in the field of artificial intelligence, where it is claimed for over 80 years that AGI will arrive “in 5 years”
And we do not have to look further from Zagreb for example where robotaxis, which – in a city lacking plaster, kindergartens, and leak-proof water pipes – are burning 200 million public euros.
When we put aside the pre-puberty statements that robot taxis will provide transportation for everyone, including schoolchildren (because obviously, first-graders should be the guinea pigs for robots); within the state NPOO document, it proudly states that fully autonomous driving is “largely ready,” which is such a big lie that the top officials from DORH should have already sharpened their pencils.
Don’t worry, AI gurus are just warming up
Fortunately, a significant portion of AI development is focused on supporting human labor, which brings us back to the main question of this text – how should we, as developers, designers, freelancers, agency workers, or product managers, look at AI and our potential daily routine?
An older economic dogma asserts that augmenting labor has greater benefits than merely replacing people. A recent GitHub study sheds light on the details – with the help of Copilot, engineers completed a test task 55% faster than their colleagues who did not have access to Copilot.
Tools like Copilot help complete tasks about 10% faster and maintain the beloved “zone” for 73% of respondents – leaving engineers with more time for deepening knowledge, improving code quality, and much-needed collaboration with UX colleagues, as Nenad has already written about.
Check out the Twitter thread that demonstrates the birth of an entirely new category of storytelling. Artificial intelligence has also started to be used for crocheting “cursed” patterns or generating endless episodes of Seinfeld. Also, Antonija and Marko have written about the ubiquitous trend of creating new types of online searches; all of these are signs of expanding creative and online industries.
The deepening and expansion of the industry due to technological advances are described in economics through Jevons’ Paradox. It states that the demand for resources increases with the introduction of new tools and efficiency, which means that the need for developers, copywriters, and designers could grow; but also that GPT gurus will not be going on vacation anytime soon.
Jevons implies embracing new tools (do you know an accountant who doesn’t know Excel?), but the sensible use of technology is the last point that we, as industry leaders, need to be louder about its real capabilities. Artificial intelligence fundamentally does not demonstrate signs of human cognition – a sufficiently large neural network will be able to imitate what people currently do to some extent, but it will not be able to conceive everything that can be done.
Imitating our brains is therefore pointless, as the most crucial and useful AI features are already tied directly in computers. Admittedly, the new fascinating moment is that our tools have become intelligent and sometimes even ingenious overnight; what is becoming clear is that those who use AI systems will have to be both. Through artificial approaches, tools, and technologies, we have managed to rise above what is possible – that is, we ourselves have become wiser, more creative, and smarter, both in the physical and virtual world.